Research

At the Spectroscopy and AI Lab (SAIL) led by Professor Joonyoung F. Joung, we are pioneering the integration of artificial intelligence with chemistry to solve complex problems in reaction prediction and new material discovery. Our approach combines experimental techniques, computational modeling, and machine learning to accelerate the development of innovative chemical solutions.
국민대학교 응용화학부 SAIL 연구실(Spectroscopy and AI Lab)에서는 인공지능과 화학 연구를 융합하여 신소재 개발을 가속화하고 화학 반응 예측을 향상시키는 데 집중하고 있습니다.
Prediction of Physicochemical Properties
We leverage machine learning models and quantum chemical calculations (e.g., DFT) to predict critical physicochemical properties of molecules, such as optical properties, energy levels, and solubility. These predictions not only aid in discovering desired materials or pharmaceuticals but also support the optimization of separation processes by providing insights into solubility behavior.
머신러닝 모델과 양자화학 계산(예: DFT)을 활용하여 분자의 광학적 특성, 분자의 에너지 준위나 용해도와 같은 물리화학적 특성을 예측합니다. 이러한 예측은 신소재나 약물을 개발하는 데 도움을 줄 뿐만 아니라, 용해도에 대한 통찰을 제공함으로써 분리 공정의 최적화에도 기여할 수 있습니다.
Selected publications:
[1]
"Deep Learning Optical Spectroscopy Based on Experimental Database: Potential Applications to Molecular Design"
Joonyoung F. Joung, Minhi Han, Jinhyo Hwang, Minseok Jeong, Dong Hoon Choi, Sungnam Park, JACS Au, 1, 427 (2021) (Link)
[2]
"Deep learning for development of organic optoelectronic devices: Efficient prescreening of hosts and emitters in deep-blue fluorescent OLED"
Minseok Jeong, Joonyoung F. Joung, Jinhyo Hwang, Minhi Han, Chang Woo Koh, Dong Hoon Choi, Sungnam Park, npj Compt. Mater. , 8, 147 (2022) (Link)
[3]
"Beyond Woodward–Fieser Rules: Design Principles of Property-Oriented Chromophores Based on Explainable Deep Learning Optical Spectroscopy"
Joonyoung F. Joung, Minhi Han, Minseok Jeong, Sungnam Park, J. Chem. Inf. Model., 62, 2933 (2022) (Link)
[4]
"Reliable experimental method for determination of photoacidity revealed by quantum chemical calculations"
Joonyoung F. Joung, Minseok Jeong, Sungnam Park, Phys. Chem. Chem. Phys., 24, 21714 (Link)
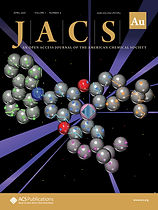
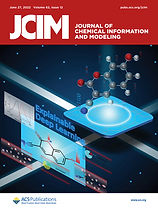
[1]
"Electron flow matching for generative reaction mechanism prediction obeying conservation laws"
Joonyoung F. Joung, Mun Hong Fong, Nicholas Casetti, Jordan P. Liles, Ne S. Dassanayake, Connor W. Coley,
arXiv preprint (2025) (Link)
Chemical Reaction Prediction
Chemical reaction prediction has traditionally relied on expert knowledge and reaction rules, making it difficult to anticipate unexpected byproducts or impurities. We develop models that predict products either in an end-to-end manner or by learning mechanistic pathways. This helps to explain impurity formation and ultimately discover novel reactions.
화학 반응 예측은 지금까지 화학자의 경험에 의존해 왔으며, 예기치 못한 생성물이나 불순물은 반복 실험으로 확인해 왔습니다. 본 연구실은 머신러닝 기반 예측 모델을 통해 반응물로부터 생성물을 직접 예측하거나, 메커니즘 수준에서 반응 경로를 학습하도록 하여 불순물 형성 원인을 설명하고 새로운 반응 발견으로 확장하는 것을 목표로 합니다.
Selected publications:
[2]
"Reproducing Reaction Mechanisms with Machine-Learning Models Trained on a Large-Scale Mechanistic Dataset"
Joonyoung F. Joung, Mun Hong Fong, Jihye Roh, Zhengkai Tu, John Bradshaw, Connor W. Coley, Angew. Chem. Int. Ed., 63, e202411296 (2024) (Link)
De Novo Molecular Design
Traditional approaches to molecular design rely heavily on trial-and-error experimentation, even when the desired properties are known. At SAIL, we develop machine learning-based de novo design techniques that generate candidate molecules expected to exhibit target properties. This accelerates material discovery while reducing cost and effort.
In addition to generative modeling, we leverage quantum chemical methods such as DFT to understand how molecular structure determines properties. This allows us to extract design principles grounded in theory and propose new molecular structures with improved performance.
기존의 분자 설계는 원하는 특성을 만족하는 분자를 직접 설계하기 어려워 반복 실험에 의존해 왔습니다. 본 연구실은 머신러닝을 활용해 목표 특성을 만족할 수 있는 분자 구조를 자동으로 생성하는 de novo 설계 모델을 개발하여, 후보 물질 탐색의 효율을 극대화합니다.
또한, 단순한 생성 모델을 넘어서 DFT 등 양자화학계산 방법을 통해 분자의 구조가 특성에 미치는 영향을 분석하고, 이론적으로 정립된 설계 원리를 도출함으로써 새로운 구조를 제안할 수 있는 기반을 마련하고 있습니다
Selected publications:
[1]
"Generative deep learning-based efficient design of organic molecules with tailored properties"
Minhi Han, Joonyoung F. Joung, Minseok Jeong, Dong Hoon Choi, Sungnam Park, ACS Cent. Sci. (Link)
[2]
"Rational Molecular Design of Azaacene-based Narrowband Green-emitting Fluorophores: Modulation of Spectral Bandwidth and Vibronic Transitions"
Jung Min Ha, Hye Beom Shin, Joonyoung Francis Joung, Won Jae Chung, Ji-Eun Jeong, Sangin Kim, Seon Hyoung Hur, Suk-Young Bae, Jun-Yun Kim, Jun Yeob Lee, Sungnam Park, Han Young Woo, ACS Appl. Mater. Interfaces, 13, 26227 (2021) (Link)
[3]
"A new visible light triggered Arrhenius photobase and its photo-induced reactions"
Joonyoung F. Joung, Jeeun Lee, Joungin Hwang, Kihang Choi, Sungnam Park, New J. Chem., 44, 668 (2020) (Link)

Database Development
Reliable AI models require high-quality data. We build structured databases by extracting information from experiments and literature, and use these data for training and benchmarking models.
인공지능 연구를 위한 고품질 데이터는 필수적입니다. 본 연구실은 화학 실험 및 문헌에서 화학 정보를 수집·정리하여 데이터베이스를 구축하고, 이를 통해 모델 학습과 새로운 소재 발견의 기반을 마련합니다.
Selected publications:
[1]
"Experimental database of optical properties of organic compounds"
Joonyoung F. Joung, Minhi Han, Minseok Jeong, Sungnam Park, Sci. Data, 7, 295 (2020) (Link)